The Importance of Balance
Last week, I talked about the election of a Doge, the Venetian empire's chief executive officer (CEO). We saw how this electoral process was a dance between choice and chance, with human desires pushing it forward, only to be modified by a throw of the dice. The underlying principle here is balance. The Venetians wanted to ensure that no single interest or family dominated the polity. They achieved this through the use of a unique downsampling technique.
The principle of balance holds in statistics and machine learning too. Because one rarely has the time or money to poll everyone in a specific population, sampling is used instead. The key idea here is simple: a sample ought to be representative of the population being studied. However, that ideal can only be achieved if the sampling is balanced. Here’s a concrete example that illustrates that idea.
In the 1936 presidential election, Alf Landon, the republican governor of Kansas, faced off against President Franklin Roosevelt. To predict how the election might turn out, the Literary Digest sent out 10 million “straw” ballots. Potential voters returned 2.4 million ballots, a return rate of approximately 25%. The results were unambiguous. The Digest predicted that Landon would beat Roosevelt 57% to 43%. As it turned out, Roosevelt won 62% of the vote to Landon’s 37%.
So, what happened? The problem was that the Literary Digest used lists of phone numbers, driver registrations, and country club memberships to create its mailing list. But in 1936, America was in the midst of the Great Depression and only the wealthy could afford luxury items like these. The vast majority of Americans had nothing. They were just trying to get by. Hence, an unbalanced sampling process resulted in an unbalanced sample, resulting in a skewed prediction.
It appears that balance is still an issue. A poll by the Des Moines Register – taken just days before the election – showed Vice President Harris leading Donald Trump 47% to 44%. But Trump beat Harris by 14 points in Iowa. It wasn’t even close. J. Ann Selzer, the renowned pollster who conducted the poll, now says she will review the data. My guess is that this was an unbalanced sample, with too many Democrats on the call list and too few Republicans.
Balance is also important in AI. A balanced training dataset, for example, is critical to success. Let’s consider a concrete example.
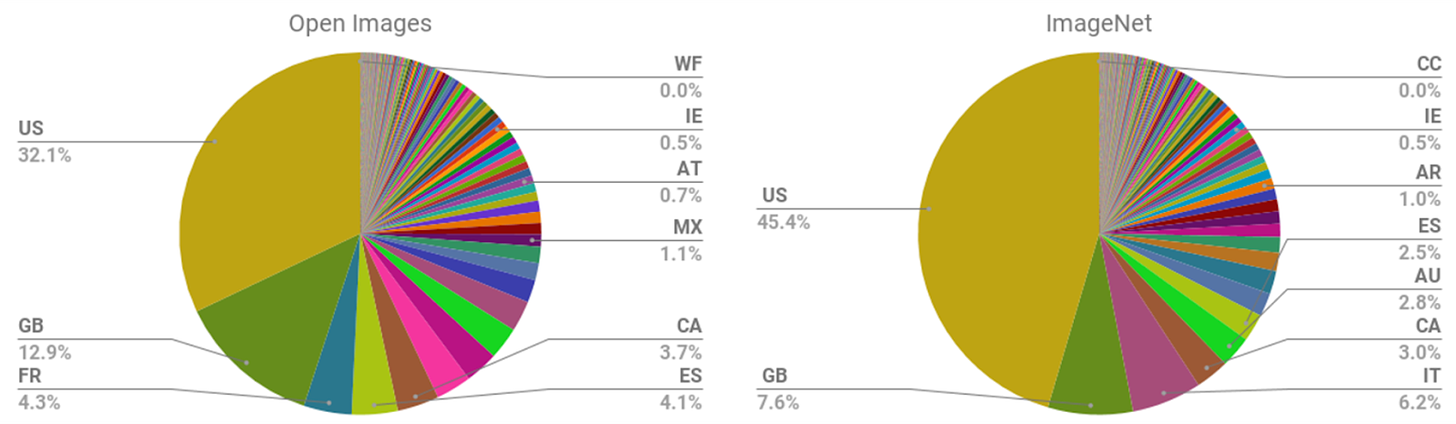
The Open Images and ImageNet training datasets have played an important role in AI over the last decade. Some of the most prestigious AI image classification contests feature these datasets in their competitions. These two pie charts identify the origin of the images in these datasets by country. But do you notice something odd? Do you see it? A majority of the images in these two datasets come from Western countries – the United States (US), Great Britain (GB), France (FR), Canada (CA), Australia (AU), and Italy (IT). But how do you think models trained on this data performed when asked to classify an image from a non-Western country or culture?
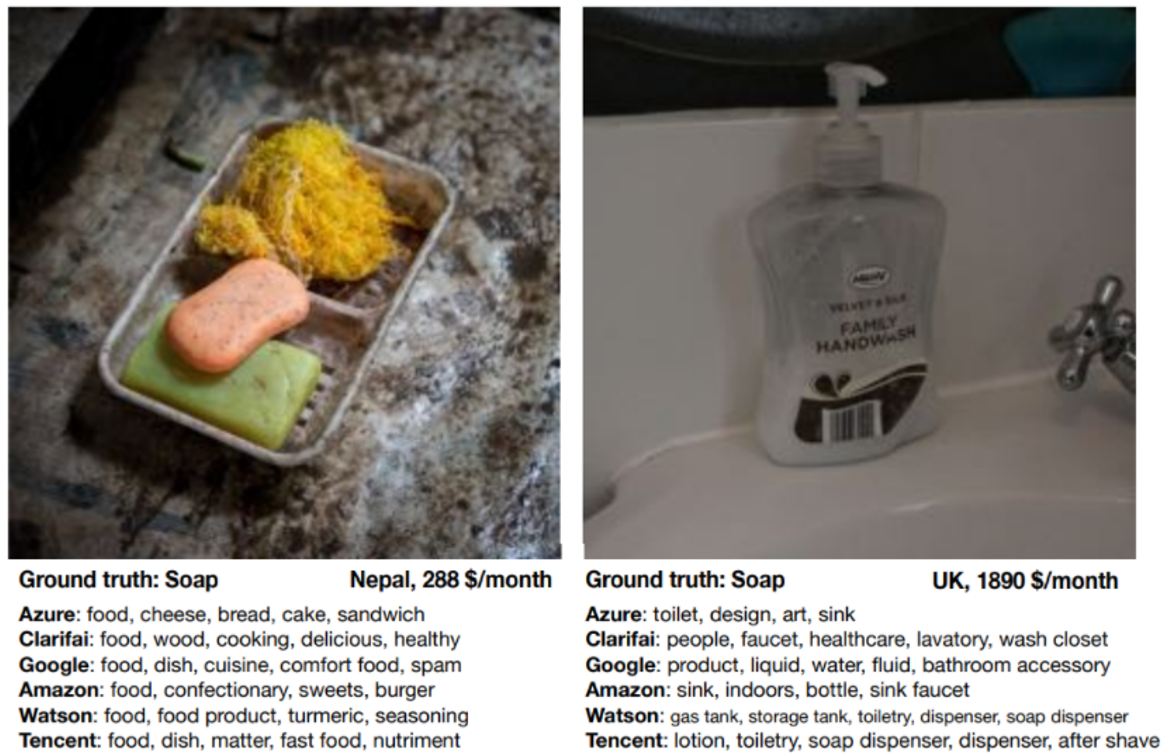
Here, we see two images. The ground truth, in this case, is soap. But look closely at what the models predicted on the left. All of them predicted that this was food or a food item. Note: these models were developed by major AI players – Google, IBM, Microsoft, and Tencent. What’s going on here? Again, it’s all about balance. Models trained on unbalanced data sets have much greater difficulty identifying household items (soap, spices, sofas, beds, and so forth) when the image in this example is from a lower-income country. In short, the models misclassified this image because they had not “seen” enough images like it during training.
We have now traversed the centuries, from the fascinating process of electing a Venetian Doge to AI training datasets. In each example, we saw that balance is a crucial concept when modeling reality. Achieving it results in a helpful model, an accurate approximation of reality. But when balance is absent, it’s like driving down the highway on a set of unbalanced tires. Everything shakes and rattles in the most uncomfortable way. When that happens, all one can do is pull over and have a tire technician add lead weights in the right places.